Michael Richardson
November 2019
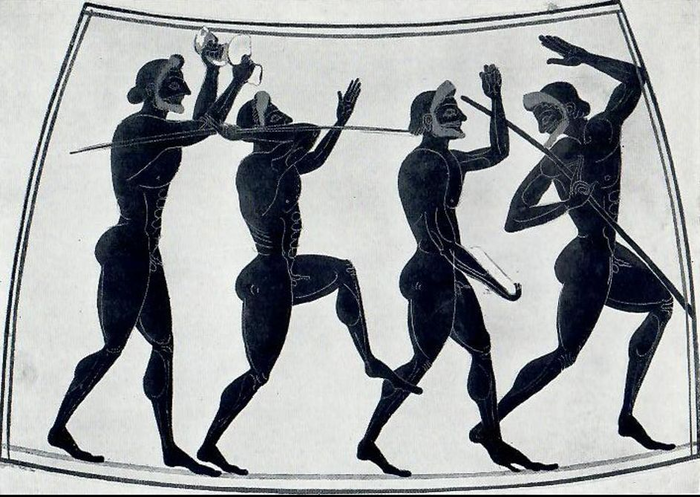
Consider the spear thrower, the archer, the rifleman: a distant target is sighted, its movements tracked, a calculation made, a missile launched. Each step is one element in a kinetic embodied process, but also a prosthetic one. Practice, instinct, and techné combine to render death at a distance. Siege engines, artillery, ballistic missiles, and aerial bombers work in much the same way. Target, calculation, missile. Technological developments have seen each of these stages intensified. Targets can be identified at great distance; calculations can be completed by computer; missiles can travel between continents with considerable accuracy. Each intensification extended the distance at which war can be waged while reducing the time between decision-making and effect. And it did so by making killing increasingly computational.
Afghanistan, Yemen, Somalia, Pakistan. A wanted figure, someone prominent on the American list of High Value Targets, is positively identified, tracked to a location, monitored, and then assassinated at a timely moment by a Hellfire missile launched from a loitering Predator drone. Like all killing at a distance, this act is embodied, prosthetic, and kinetic: the drone enables the vision of operators to extend across thousands of kilometres, bringing distant war into mediated contact with operators located in ground control stations full of screens and interfaces. Computation is key. Vision is binary, ones and zeroes transmitted by satellite and cables and rendered into pixels visible to operators. Drones are launched and landed by intelligent autopilot. Missile trajectories are mapped, tracked, and maintained by microprocessors, sensors, and signals. The difference in all this from the spear is one of degree, not kind: the fundamental process and its underlying logic remain in place.
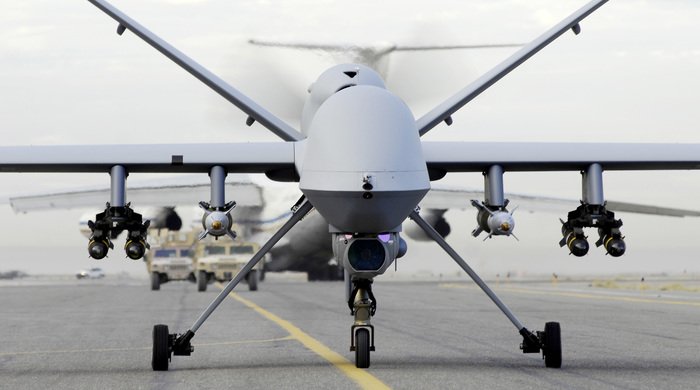
Popular imaginaries of drone warfare cohere around exactly this precision killing of specific individuals. Yet these ‘personality strikes’ are far outnumbered by ‘signature strikes’ in their actual occurrence. Signature strikes depend on ‘pattern of life analysis’ (Gregory 2011; Chamayou 2013), which seeks to map sustained patterns in daily rhythms and activities in order to identify traces of a potential enemy. Phone calls, spatial movements, financial transactions, meetings: collected, logged, mapped, and processed according to an opaque set of criteria and algorithmic operations, pattern of life analysis generates threats from disparate data. What Joseph Pugliese (2016) calls ‘death by metadata’ leaves ruined bodies, shattered communities, fractured worlds. This is death predicated on prediction: on the calculated expectation that a particular set of data points authenticates threat, verifies its presence in the same way that a practised gesture of the hand makes the claim that I am me. Here in the signature strike we find difference in kind: killing because of what might come to pass, what might come to be, who someone becomes within the logic of the data, and according to the algorithm.
Signature strikes depend on the generation of threat. People are killed because they might have been threats if they were allowed to live. ‘Threat is from the future’, writes affect theorist Brian Massumi (2010, 53). So too, it seems, is algorithmic killing. No particular person is targeted in any signature strike: it is the signature of what might come to pass that produces death. The rendering of facets of daily life into data – movements of cell phones, patterns of calls and texts, attendance at gatherings, relations of acquaintance or family or clan – produces signs of what might be if the future unfurls unchecked. Potentiality shifts from the ethereal to the material: what might be becoming what is without the person on the ground ever knowing. This is lethal force exercised at a distance and predicated on prediction. It is what Massumi (2015) calls ‘ontopower’: power over being and to bring into being, manifested in the emergence from computational systems into the most absolute of sovereign decisions.
There is an elaborate infrastructure of predictive killing: remote sensors, signal transmitters and receivers, algorithmic processes, data storage centres, encryption encodings, image analysis practices, human-computer interactions, rules of engagement, military laws and codes. The kill chain – the interlinked procedure for the authorisation of life, the enactment of the sovereign decision – ties the data-driven algorithmic prediction and the drone’s capacity to perceive to its power to inflict death. Drone killing entangles law, too, even as it exceeds or fractures its boundaries and workings. Yet for all its complexity the apparatus of predictive killing grinds against what it can know about the world. Pattern of life analysis cannot avoid what Paul Edwards (2010), in his account of climate monitoring regimes, calls data friction: the innumerable points at which the very collection of data rubs against accuracy, prevents clear attribution, introduces uncertainty into the system. Cell towers break down, images are incorrectly processed, errors of translation abound.
Writing on its embodied and embodying dynamics, Lauren Wilcox (2017) points out that ‘drone warfare simultaneously produces bodies in order to destroy them, while insisting on the legitimacy of this violence through gendered and racialized assumptions about who is a threat’ (21). In this sense, then, drone warfare is necropolitical. The transgression of boundaries through systems of calculation, measurement, and instrumentality described by Achille Mbembe (2003) are enacted in drone warfare’s management of life and death, its production of certain bodies as threatening such that they must be killed. But it is not only calculated rationality at work here: it is the instrumentalisation of machinic prediction that enables slaughter.
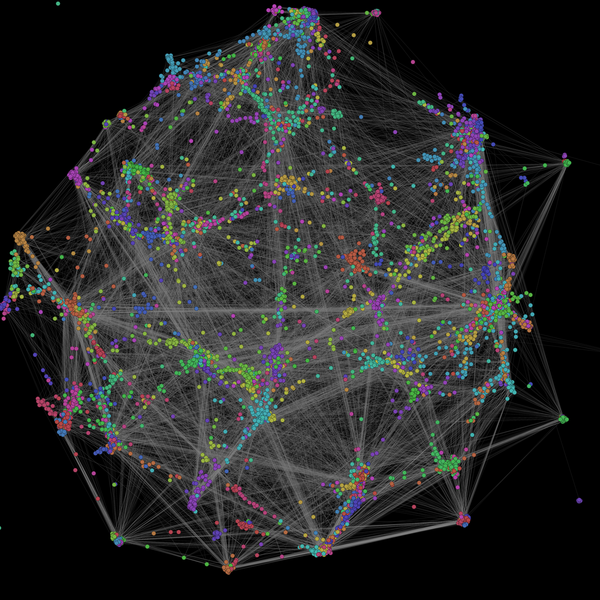
Adrian Mackenzie (2015) writes that algorithmic ‘prediction depends on classification, and classification itself presumes the existences of classes, and attributes that define membership of classes’ (433). More, it requires the relative stability of classifications, such that any given object stays within its class. What, then, does the data coding and algorithmic analysis – or indeed the human analysis – make of the fluid status afforded to all sorts of bodies and activities in the borderlands subjected to drone surveillance and killing? Drone vision and the aerial images it generates are not easy to decode, even for the most highly trained human operator. Is the cylindrical object on a figure’s shoulder a rocket launcher or a rolled-up prayer rug? Is a cluster of bodies inside a home – sensed with a thermal camera and rendered as a lump of white – a tribal meeting, a family meal or a terrorist cell? Has a phone been loaned from one brother to another, a sim card passed around a village, a vehicle borrowed for a long journey? Who might be an important elder in one context, a militant in another, a father in a third? These instances of slippage in status are not simple errors but proliferating uncertainties that threaten to undermine the very system itself – if, that is, its infrastructures were not so unequal.
Drone warfare depends upon an absolute inequality: its infrastructures constitute, reproduce and rely on the certainty that the racialised and gendered bodies that are its targets, both immediately and potentially, can never invert the lethal paradigm. Identifying or erasing the data collected about us by social media platforms, credit rating companies, and government agencies might prove futile, but there are at the very least points of potential access to those entities. Nothing like this exists for peoples living under drones across the global south, in the proliferating zones of America’s perpetual war, the occupation of Palestine or the Russian airbases in Syria. False positives leave ruined flesh in their aftermath – and the algorithms themselves don’t seem to learn from these results. To have been killed, the threat must have been real: after all, it was made manifest in the data, advocated by algorithm, executed via computation, sensor, software – and by human decision. Only in the most extreme cases – the convoy of women and children, say – do false positives enter the system. And, in any case, who among us can tell how the infrastructure shifts, the algorithms learn, the data becomes less frictive?
The opacity of algorithms, the secrecy of institutions, the inaccessibility of evidence, the frictions of data: these problems are pushed to the limit in predictive killing but are by no means unique to drone war. Algorithmic infrastructures always entail precisely these concerns: consider, for instance, the controversies surrounding Facebook’s algorithms and their exploitation for political purposes. But for predictive infrastructures – policing tools such as Predpol, judicial sentencing instruments like Compass, the credit ratings of Experian and other companies, even the search rankings of Google – these problematics intersect and multiply precisely because of their orientation towards the future. Because predictive algorithms produce action in the present on the yet-to-arrive, they produce the future itself – virtually and provisionally yet nonetheless actually acted upon.
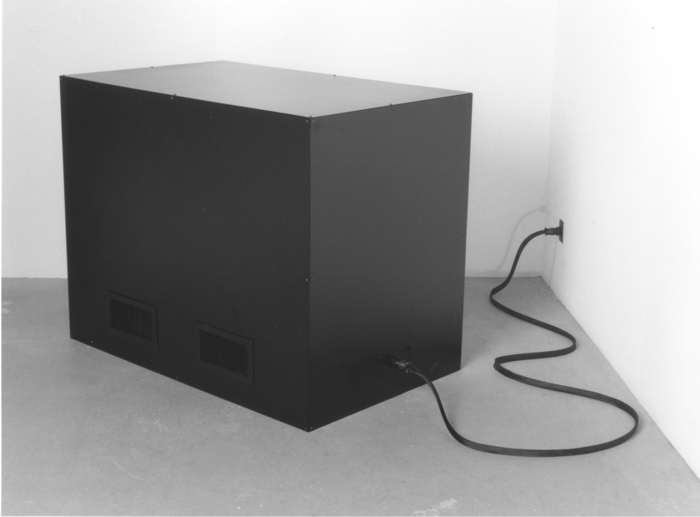
What, then, is to be done?
The opacity of algorithms lurks within the language used to describe them: a step-by-step instruction of how to solve a task, a recipe, a form of programmed logic, an automated filtering mechanism, code, magical (Finn 2017). Algorithms resist clear explanation precisely because they are operative and transformative: computational alchemy. As Nick Seaver (2013) writes, ‘in spite of the rational straightforwardness granted to them by critics and advocates, “algorithms” are tricky objects to know’ (2), such that ‘a determined focus on revealing the operations of algorithms risks taking for granted that they operate clearly in the first place’ (8). Yet public debate and academic inquiry too often concern what takes place within algorithms as a means of exposing the inequalities they produce, support, elide, and rely on. What is needed, first and foremost, is an emphasis with what algorithmic infrastructures do.
Algorithms make the world appear one way and not another: predictive algorithms project worldly appearance into the future. Taina Bucher (2018) writes that ‘algorithmic systems embody an ensemble of strategies, where power is immanent in the field of action and situation in question’ (3). This is precisely what drives their appeal: the algorithmic seduces via its co-opting of decision. To ask what algorithms do, then, is not simply a matter of what they do to data or even the actions they enable (deny this loan, destroy that home). Algorithms serve the political function of evacuating the space of politics of the necessity of decision: algorithms appeal to the state precisely because they enable the deferral of responsibility, the wiping away of risk, the occlusion that comes with computational complexity and technological fetishism. Policymaking is pushed further from implementation, cushioned from inquiry and critique by inscrutable code.
Whether at work in killing, policing, profiling or advertising, predictive algorithms demand new lines of inquiry – academic, investigative, public, political – that shift the locus of attention from within its workings to its contacts with the world. To expose and unknot the inequalities engendered by the proliferation of algorithms within state infrastructures, we might begin with new questions:
What are its discursive, affective and material functions? How does it come into contact with bodies, how does it sense, know, shape, and produce them?
What value does the algorithm, predictive or otherwise, offer its political masters? What elisions, deferrals, and evasions of responsibility? What injustices does it hide?
Upon whom does the algorithm act? To whose benefit? And with what points of intervention or access for those entangled in the system?
What are the points of friction and the failures of capture in the extraction of data?
How stable are its classifications? Or, rather, how certain are the foundations upon which prediction is predicated?
What are the aesthetics and politics of its modes of representation? What is precluded, included or excluded from its manifestation in forms accessible and knowable to the human?
Consider the predictive algorithm, then, not simply as technology, actualised in action like the javelin hurled towards its target. Consider the algorithm as on the brink of fragility, exposed to uncertainty and inherently provisional. For all its power and political potency, the predictive algorithm – even the killing kind – relies upon inequalities and opacities. Its authority is fetishistic, a product of faith as much as accuracy. Chasing the algorithm into its rabbit hole of black-boxed code will be far more often futile than not. The trick of critique is not to make the algorithm transparent, but to make visible precisely what its opacity and inequality make possible.
Works Cited
Bucher, Taina. 2018. If…Then: Algorithmic Power and Politics. Oxford and New York: Oxford University Press.
Chamayou, Grégoire. 2015. Drone Theory. Translated by Janet Lloyd. London: Penguin.
Edwards, Paul N. 2010. A Vast Machine: Computer Models, Climate Data, and the Politics of Global Warming. Cambridge, MA: MIT Press.
Finn, Ed. 2017. What Algorithms Want: Imagination in the Age of Computing. Cambridge, MA: MIT Press.
Gregory, Derek. 2011. ‘From a View to a Kill: Drones and Late Modern War.’ Theory, Culture & Society, 28 (7–8): 188—215.
Massumi, Brian. 2015. Ontopower: War, Powers, and the State of Perception. Durham, NC: Duke University Press.
Massumi, Brian. 2010. ‘The Future Birth of the Affective Fact: The Political Ontology of Threat.’ In M. Gregg and J. Seigworth (eds) The Affect Theory Reader, 52—70. Durham, NC: Duke University Press.
Mbembe, Achille. 2003. ‘Necropolitics.’ Public Culture, 15 (1): 11—40.
Pugliese, Joseph. 2016. ‘Death by Metadata: The Bioinformationalisation of Life and the Transliteration of Algorithms to Flesh.’ In H. Randell-Moon and R. Tippet (eds) Security, Race, Biopower: Essays on Technology and Corporeality, 3—20. London: Palgrave Macmillan.
Seaver, Nick. 2013. ‘Knowing Algorithms.’ Media in Transition 8. Cambridge, MA.
Wilcox, Lauren. 2017. ‘Embodying Algorithmic War: Gender, Race, and the Posthuman in Drone Warfare.’ Security Dialogue, 48 (1): 11—28.